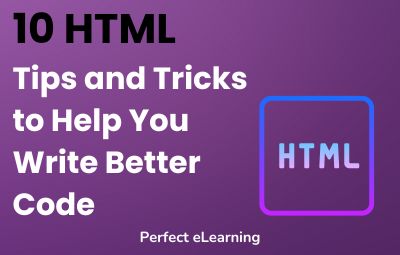

As the field of data science continues to grow and evolve, there is increasing recognition of the critical role that statistics plays in extracting insights and making informed decisions from data.
Understanding Statistics in Data Science
At its core, statistics is a branch of mathematics that deals with the collection, analysis, interpretation, presentation, and organization of data. In the context of data science, statistics provides the tools and methods for making sense of complex and often large datasets. By using statistical techniques, data scientists can identify patterns and trends, test hypotheses, and make predictions about future outcomes.
Key Statistical Techniques in Data Science
Some of the key statistical techniques used in data science include:
Descriptive Statistics
Descriptive statistics is the branch of statistics that deals with summarizing and describing the main features of a dataset. This includes measures of central tendency (such as mean, median, and mode), measures of spread (such as standard deviation and range), and measures of shape (such as skewness and kurtosis). Descriptive statistics is often used as a first step in data exploration and visualization.
Inferential Statistics
Inferential statistics is the branch of statistics that deals with making inferences about a population based on a sample of data. This includes hypothesis testing, which is used to determine whether an observed effect is statistically significant, and confidence intervals, which provide a range of values within which a population parameter is likely to fall.
Regression Analysis
Regression analysis is a statistical technique that is used to model the relationship between a dependent variable and one or more independent variables. This includes linear regression, which models a linear relationship between variables, and logistic regression, which models the probability of a binary outcome.
Machine Learning
Machine learning is a branch of artificial intelligence that uses statistical techniques to enable computer systems to learn from data and make predictions or decisions without being explicitly programmed. This includes supervised learning, unsupervised learning, and reinforcement learning.
Conclusion
Statistics plays a critical role in data science, providing the foundation for many of the key techniques and tools used to extract insights and make informed decisions from data. By understanding the role of statistics in data science, businesses and organizations can better leverage the power of data to drive innovation, increase efficiency, and achieve their goals.
Frequently asked Questions (FAQs)
Q. Why is statistics important in data science?
Statistics provides the necessary tools and techniques for data scientists to make sense of complex datasets, identify patterns and trends, test hypotheses, and make predictions about future outcomes. Without a strong foundation in statistics, data science would not be as effective in providing insights and making informed decisions from data.
Q. What are some of the key statistical techniques used in data science?
Some of the key statistical techniques used in data science include descriptive statistics, inferential statistics, regression analysis, and machine learning. These techniques enable data scientists to explore and analyze data, make inferences about populations based on samples, model relationships between variables, and make predictions or decisions based on data.
Q. How can businesses leverage the power of statistics in data science?
Businesses can leverage the power of statistics in data science by using it to gain insights into customer behavior, optimize operations, improve decision-making, and drive innovation. By analyzing data and drawing conclusions based on statistical analysis, businesses can make better-informed decisions that can help them achieve their goals.
Q. What are some of the challenges associated with using statistics in data science?
Some of the challenges associated with using statistics in data science include dealing with missing or incomplete data, selecting appropriate statistical models and methods, ensuring the accuracy and reliability of data, and communicating statistical findings to non-technical stakeholders. Data scientists must be skilled in addressing these challenges in order to use statistics effectively in data science.
Q. How can individuals learn more about statistics in data science?
There are many resources available for individuals who want to learn more about statistics in data science, including online courses, books, and tutorials. It's important to seek out reputable sources and to practice applying statistical techniques in real-world contexts in order to build a strong foundation in statistics for data science.
Perfect eLearning is a tech-enabled education platform that provides IT courses with 100% Internship and Placement support. Perfect eLearning provides both Online classes and Offline classes only in Faridabad.
It provides a wide range of courses in areas such as Artificial Intelligence, Cloud Computing, Data Science, Digital Marketing, Full Stack Web Development, Block Chain, Data Analytics, and Mobile Application Development. Perfect eLearning, with its cutting-edge technology and expert instructors from Adobe, Microsoft, PWC, Google, Amazon, Flipkart, Nestle and Info edge is the perfect place to start your IT education.
Perfect eLearning provides the training and support you need to succeed in today's fast-paced and constantly evolving tech industry, whether you're just starting out or looking to expand your skill set.
There's something here for everyone. Perfect eLearning provides the best online courses as well as complete internship and placement assistance.
Keep Learning, Keep Growing
If you are confused and need Guidance over choosing the right programming language or right career in the tech industry, you can schedule a free counselling session with Perfect eLearning experts.