
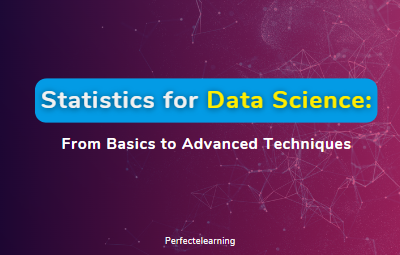
Statistics
Statistics is a field of mathematics that involves the gathering, analysis, interpretation, presentation, and organization of data. It provides a framework for understanding, modeling, and predicting phenomena that involve uncertainty and variability. In data science, statistics is used to extract insights from data, build models, and make decisions.
Descriptive Statistics
Descriptive statistics is the branch of statistics that deals with summarizing and visualizing data. It includes measures of central tendency, such as mean, median, and mode, and measures of dispersion, such as variance, standard deviation, and range. Descriptive statistics can be used to explore and understand data, identify patterns, and detect outliers.
Inferential Statistics
Inferential statistics is the branch of statistics that deals with making inferences about a population based on a sample. It involves hypothesis testing, confidence intervals, and estimation. Inferential statistics can be used to test hypotheses, make predictions, and generalize findings to the population.
Probability Distributions
Probability distributions are mathematical functions that describe the likelihood of different outcomes in a random process. They are used to model and analyze data in many fields, including finance, physics, engineering, and biology. Some commonly used probability distributions in data science include the normal distribution, binomial distribution, and Poisson distribution.
Hypothesis Testing
Hypothesis testing is a statistical method that is used to test a hypothesis about a population based on a sample. It involves defining a null hypothesis, which is the assumption that there is no difference between two groups, and an alternative hypothesis, which is the assumption that there is a difference. Hypothesis testing can be used to determine if a result is statistically significant, which means that it is unlikely to have occurred by chance.
Regression Analysis
Regression analysis is a statistical technique employed to establish a model describing the correlation between a dependent variable and one or more independent variables. It helps to forecast the values of the dependent variable by considering the values of the independent variables. Regression analysis can be used to analyze trends, make predictions, and identify outliers.
Time Series Analysis
Time series analysis is a statistical method that is used to analyze data that is collected over time. It involves modeling the time-dependent behavior of a variable and identifying patterns, trends, and seasonal effects. Time series analysis can be used to make predictions and identify anomalies in time series data.
Clustering Techniques
Clustering techniques are used to group data points into clusters based on their similarity. They are used to identify patterns and structure in data, and to segment customers, products, or services. Some commonly used clustering techniques in data science include k-means clustering, hierarchical clustering, and density-based clustering.
Principal Component Analysis
Principal Component Analysis (PCA) is a statistical technique utilized to decrease the complexity of data by reducing its dimensionality. It involves transforming the data into a new set of variables, called principal components, which capture the maximum amount of variance in the original data. PCA can be used to visualize high-dimensional data, identify patterns, and reduce noise.
Conclusion
Statistics is a crucial tool in data science, as it provides a framework for understanding and analyzing data. In this article, we covered the basics of statistics and gradually moved towards the advanced techniques that data scientists use, such as regression analysis, time series analysis, clustering techniques, and principal component analysis.By mastering the statistical techniques covered in this article, data scientists can extract insights from data, build models, and make data-driven decisions. However, it's important to note that statistics is not a one-size-fits-all solution, and the choice of technique depends on the nature of the data and the problem at hand.In conclusion, statistics is a fundamental component of data science, and data scientists must have a solid understanding of the basic concepts and techniques to be effective in their work.
FREQUENTLY ASKED QUESTIONS (FAQs)
Q.What is the difference between descriptive and inferential statistics?
A. Descriptive statistics deals with summarizing and visualizing data, while inferential statistics deals with making inferences about a population based on a sample.
Q.What is hypothesis testing, and why is it important?
A. Hypothesis testing is a statistical method that is used to test a hypothesis about a population based on a sample. It's important because it allows us to determine if a result is statistically significant, which means that it is unlikely to have occurred by chance.
Q.What is principal component analysis, and how is it used in data science?
A. Principal component analysis is a statistical method that is used to reduce the dimensionality of data. It's used in data science to visualize high-dimensional data, identify patterns, and reduce noise.
Q.What are some commonly used clustering techniques in data science?
A. Some commonly used clustering techniques in data science include k-means clustering, hierarchical clustering, and density-based clustering.
Perfect eLearning is a tech-enabled education platform that provides IT courses with 100% Internship and Placement support. Perfect eLearning provides both Online classes and Offline classes only in Faridabad.
It provides a wide range of courses in areas such as Artificial Intelligence, Cloud Computing, Data Science, Digital Marketing, Full Stack Web Development, Block Chain, Data Analytics, and Mobile Application Development. Perfect eLearning, with its cutting-edge technology and expert instructors from Adobe, Microsoft, PWC, Google, Amazon, Flipkart, Nestle and Infoedge is the perfect place to start your IT education.
Perfect eLearning provides the training and support you need to succeed in today's fast-paced and constantly evolving tech industry, whether you're just starting out or looking to expand your skill set.
There's something here for everyone. Perfect eLearning provides the best online courses as well as complete internship and placement assistance.
Keep Learning, Keep Growing.
If you are confused and need Guidance over choosing the right programming language or right career in the tech industry, you can schedule a free counselling session with Perfect eLearning experts.