
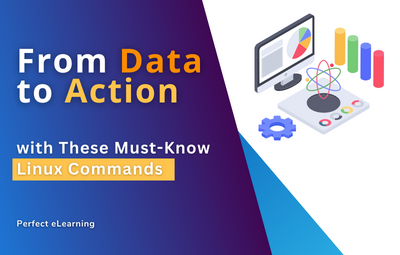
In recent years, the amount of data generated by businesses has skyrocketed due to technological advancements and the widespread use of the internet. This data explosion has presented both opportunities and challenges for enterprises across various industries. To stay competitive and thrive in this data-rich landscape, businesses must be able to efficiently manage, process, and analyze vast amounts of data. This is where data engineering comes into play.
2. What is Data Engineering?
2.1 Definition and Purpose
Data engineering can be defined as the process of designing, developing, and maintaining systems that collect, transform, and store data for analysis and decision-making purposes. The primary purpose of data engineering is to enable organizations to turn raw data into actionable insights.
2.2 Key Components of Data Engineering
Data engineering involves several key components, including data ingestion, data transformation, data storage, and data retrieval. Each of these components plays a crucial role in the overall data engineering process.
3. The Role of Data Engineering in Business Transformation
3.1 Data-Driven Decision Making
One of the most significant advantages of data engineering is its ability to facilitate data-driven decision making. By processing and analyzing data in real-time, businesses can gain valuable insights into customer behavior, market trends, and operational performance.
3.2 Enhancing Customer Experience
Data engineering allows companies to collect and analyze customer data, enabling them to personalize products and services according to individual preferences. This personalization enhances the overall customer experience and fosters customer loyalty.
3.3 Optimizing Operations and Efficiency
By harnessing the power of data, businesses can optimize their operations and improve efficiency. Data engineering enables the integration of various data sources, allowing companies to identify bottlenecks, streamline processes, and reduce costs.
3.4 Predictive Analytics and Machine Learning
Data engineering forms the foundation for predictive analytics and machine learning models. These advanced analytics techniques enable businesses to forecast trends, detect anomalies, and make accurate predictions, empowering them to make proactive decisions.
4. Data Engineering Tools and Technologies
4.1 Data Integration Tools
Data integration tools allow businesses to consolidate data from diverse sources into a unified platform. These tools facilitate smooth data flow and ensure data accuracy.
4.2 Data Warehousing Solutions
Data warehousing solutions provide a centralized repository for storing structured and unstructured data. These warehouses enable easy data retrieval for analysis and reporting purposes.
4.3 Big Data Processing Frameworks
Big data processing frameworks like Apache Hadoop and Spark enable businesses to process and analyze vast datasets efficiently. These frameworks can handle both structured and unstructured data, making them ideal for handling diverse data types.
5. Challenges in Data Engineering
5.1 Data Quality and Consistency
Ensuring data quality and consistency is a significant challenge in data engineering. Inaccurate or inconsistent data can lead to faulty analyses and misguided decision making.
5.2 Data Security and Privacy
As data becomes more valuable, ensuring its security and privacy becomes crucial. Data engineering must implement robust security measures to protect sensitive information from breaches and unauthorized access.
5.3 Scalability and Performance
As data volumes continue to grow, data engineering solutions must be scalable and performant. Ensuring that systems can handle increasing data loads is essential for seamless operations.
6. Best Practices in Data Engineering
6.1 Data Governance and Management
Implementing strong data governance and management practices is essential for data engineering success. Establishing data standards, policies, and guidelines helps maintain data integrity and reliability.
6.2 Data Pipeline Automation
Automating data pipelines streamlines the data engineering process and reduces manual intervention. Automation improves efficiency and reduces the risk of errors.
6.3 Continuous Monitoring and Improvement
Data engineering is an ongoing process, and continuous monitoring is essential to identify and rectify issues promptly. Regular improvements based on feedback and analysis ensure data engineering remains effective.
7. The Future of Data Engineering
7.1 Artificial Intelligence and Automation
Artificial intelligence and automation will play an increasingly significant role in data engineering. AI-powered systems can enhance data processing speed and accuracy.
7.2 Edge Computing and IoT
Edge computing and the Internet of Things (IoT) will generate vast amounts of data at the network's edge. Data engineering will be crucial in processing and analyzing this data in real-time.
7.3 Democratization of Data
Data engineering will empower businesses to democratize data access, allowing employees at all levels to make data-driven decisions independently.
8. Conclusion
Data engineering is at the heart of the data revolution, transforming how businesses operate and make decisions. By harnessing the power of data, companies can gain a competitive edge and drive innovation. As technology continues to advance, data engineering will continue to shape the future of businesses across the globe.
FAQs(Frequently Asked Questions)
Q1. What is data engineering?
A1: Data engineering is the process of designing, developing, and maintaining systems that collect, process, and store data for analysis and decision-making purposes.
Q2. How does data engineering enhance customer experience?
A2: Data engineering enables businesses to collect and analyze customer data, leading to personalized products and services that improve the overall customer experience.
Q3. What are some challenges in data engineering?
A3: Challenges in data engineering include ensuring data quality and consistency, maintaining data security and privacy, and addressing scalability and performance issues.
Q4. How does data engineering support predictive analytics and machine learning?
A4: Data engineering provides the foundational infrastructure for predictive analytics and machine learning models, allowing businesses to make accurate predictions and proactive decisions.
Q5. What does the future of data engineering hold?
A5: The future of data engineering will involve increased adoption of artificial intelligence, automation, edge computing, and the democratization of data access.
Perfect eLearning is a tech-enabled education platform that provides IT courses with 100% Internship and Placement support. Perfect eLearning provides both Online classes and Offline classes only in Faridabad.
It provides a wide range of courses in areas such as Artificial Intelligence, Cloud Computing, Data Science, Digital Marketing, Full Stack Web Development, Block Chain, Data Analytics, and Mobile Application Development. Perfect eLearning, with its cutting-edge technology and expert instructors from Adobe, Microsoft, PWC, Google, Amazon, Flipkart, Nestle and Infoedge is the perfect place to start your IT education.
Perfect eLearning provides the training and support you need to succeed in today's fast-paced and constantly evolving tech industry, whether you're just starting out or looking to expand your skill set.
There's something here for everyone. Perfect eLearning provides the best online courses as well as complete internship and placement assistance.
Keep Learning, Keep Growing.
If you are confused and need Guidance over choosing the right programming language or right career in the tech industry, you can schedule a free counseling session with Perfect eLearning experts.