
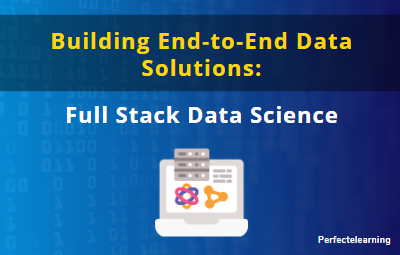
Learn about the benefits and challenges of building end-to-end data solutions with full-stack data science. Gain a competitive advantage in data-driven decision-making.
In today's data-driven world, data science has become an essential tool for businesses to make informed decisions. Data science, in simple terms, is the process of extracting insights and knowledge from data. However, the real value of data science lies in building end-to-end data solutions that can turn data into actionable insights. In this article, we will explore the concept of full-stack data science and how it can help businesses build end-to-end data solutions.
Full Stack Data Science
Full-stack data science refers to the process of creating end-to-end data solutions that encompass the entire data pipeline. It involves working with data from its raw form to its final insights and presenting it in a user-friendly format. Full-stack data science requires a wide range of skills, including data collection, data cleaning, data analysis, data visualization, and machine learning. A full-stack data scientist is someone who possesses all these skills and can work on every aspect of the data pipeline.
The Data Pipeline
The data pipeline is a series of steps that data goes through from collection to analysis. The steps involved in the data pipeline are:
1.Data Collection: Data collection is the process of gathering raw data from various sources. It can be done through various methods, including web scraping, API calls, surveys, and public datasets.
2.Data Cleaning: Data cleaning involves the process of removing noise, errors, and inconsistencies from the raw data. It is an essential step in the data pipeline as it ensures that the data is accurate and reliable.
3.Data Analysis: Data analysis is the process of using statistical and machine learning techniques to extract insights from the data. It involves identifying patterns, trends, and relationships within the data.
4.Data Visualization: Data visualization involves the process of presenting the insights obtained from data analysis in a visual format. It helps to communicate the insights effectively to stakeholders.
5.Machine Learning: Machine learning involves the process of building models that can make predictions or classify data. It is used to automate decision-making processes and provide recommendations based on the insights obtained from data analysis.
The Role of Full Stack Data Scientists
Full-stack data scientists play a crucial role in building end-to-end data solutions. They possess a wide range of skills and can work on every aspect of the data pipeline. Some of the key roles of full-stack data scientists are:
1.Data Collection and Cleaning: Full-stack data scientists are responsible for collecting and cleaning raw data from various sources. They must ensure that the data is accurate and reliable.
2.Data Analysis and Visualization: Full-stack data scientists are responsible for analyzing the data and presenting the insights obtained from it in a user-friendly format. They must communicate the insights effectively to stakeholders.
3.Machine Learning: Full-stack data scientists are responsible for building machine learning models that can make predictions or classify data. They must ensure that the models are accurate and reliable.
4.Deployment: Full-stack data scientists are responsible for deploying the end-to-end data solutions they create. They must ensure that the solutions are scalable, secure, and easy to maintain.
Tools and Technologies Used in Full Stack Data Science
Full-stack data science requires the use of various tools and technologies. Some of the popular tools and technologies used in full-stack data science are:
1.Python: Python is a popular programming language used in data science. It has a wide range of libraries and frameworks that make it easy to work with data.
2.SQL: SQL is a database language used to manage and manipulate data. It is an essential tool in data management and data analysis.
3.Tableau: Tableau is a popular data visualization tool that allows users to create interactive visualizations and dashboards.
Benefits of Full Stack Data Science
Full-stack data science has several benefits for businesses. Some of the key benefits are:
1.Improved Decision Making: Full-stack data science helps businesses make informed decisions by providing insights and recommendations based on data analysis. This helps to improve the accuracy and effectiveness of decision-making processes.
2.Increased Efficiency: Full-stack data science helps to automate processes and reduce manual effort, which increases efficiency and productivity.
3.Competitive Advantage: Full-stack data science helps businesses gain a competitive advantage by providing insights and recommendations that can be used to optimize business processes and improve customer experience.
4.Scalability: Full-stack data solutions can be scaled to handle large amounts of data, making them suitable for businesses of all sizes.
Challenges of Full Stack Data Science
Full-stack data science also has some challenges that need to be addressed. Some of the key challenges are:
1.Data Quality: Data quality is crucial in full-stack data science as it affects the accuracy and reliability of the insights obtained from data analysis. Data cleaning is a critical step in the data pipeline that helps to ensure data quality.
2.Skill Gap: Full-stack data science requires a wide range of skills, including data collection, data cleaning, data analysis, data visualization, and machine learning. It can be challenging to find individuals who possess all these skills.
3.Cost: Full-stack data science can be costly, as it requires the use of various tools and technologies, including cloud computing platforms and data visualization tools.
Conclusion
Full-stack data science is an essential tool for businesses to make informed decisions based on data analysis. It involves working with data from its raw form to its final insights and presenting it in a user-friendly format. Full-stack data scientists possess a wide range of skills and can work on every aspect of the data pipeline. However, full-stack data science also has some challenges, including data quality, skill gap, and cost. Businesses that invest in full-stack data science can gain a competitive advantage, improve decision-making processes, increase efficiency, and scale their operations.
FREQUENTLY ASKED QUESTIONS (FAQs)
Q. What is full-stack data science?
A. Full-stack data science is the process of creating end-to-end data solutions that encompass the entire data pipeline.
Q. What are the key skills required for full-stack data science?
A. The key skills required for full-stack data science include data collection, data cleaning, data analysis, data visualization, and machine learning.
Q. What are the benefits of full-stack data science?
A. The benefits of full-stack data science include improved decision making, increased efficiency, competitive advantage, and scalability.
Q. What are the challenges of full-stack data science?
A. The challenges of full-stack data science include data quality, skill gap, and cost.