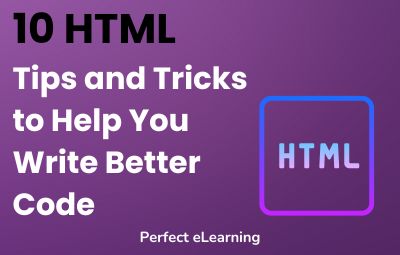

With the rise in technology, email communication has become a crucial part of our daily lives.
In 2024, the number of global e-mail users is set to grow to 4.48 billion users, up from 3.8 billion in 2018. However, with the increase in email usage, there has also been a surge in the number of unwanted emails or spam mail. These unsolicited messages can be annoying, time-consuming, and pose a significant risk to cybersecurity. To tackle this issue, researchers have been developing techniques for spam mail detection, and one of the most promising approaches is using machine learning. Here we will discuss how machine learning techniques such as natural language processing, text classification, feature engineering, and different algorithms can help in spam mail detection using machine learning.
Key steps to Spam Mail Detection:
- Email Filtering: One of the primary methods for spam mail detection is email filtering. It involves categorize incoming emails into spam and non-spam. Machine learning algorithms can be trained to filter out spam mails based on their content and metadata.
- Natural Language Processing: Natural Language Processing (NLP) is a technique that enables machines to understand and process human language. It plays a crucial role in spam detection, as it helps in extracting meaningful features from emails such as subject, body, and attachments.
- Text Classification: Text classification is a supervised learning technique used for spam detection. It involves labelling emails as spam or non-spam based on their features, such as the presence of certain keywords, tone, or grammar.
- Feature Engineering: Feature engineering is the process of selecting relevant features from the email to classify it as spam or non-spam. It involves extracting features such as the sender's email address, the presence of certain words or phrases, and the length of the email.
- Supervised Learning: Supervised learning is a technique that involves training the model on labelled data to predict the labels of new, unlabeled data. It is widely used in spam detection for text classification tasks.
- Unsupervised Learning: Unsupervised learning is a technique used to find hidden patterns in the data without the need for labelled data. It can be used for anomaly detection, clustering, and association rule mining.
- Deep Learning: Deep learning is a subfield of machine learning that involves training deep neural networks with multiple hidden layers to learn complex features from the data. It has shown great promise in spam detection tasks.
- Neural Networks: Neural networks are a type of deep learning model inspired by the human brain. They can be trained to extract meaningful features from emails and classify them as spam or non-spam.
- Decision Trees: Decision trees are a simple yet effective algorithm used for classification tasks. They can be used for feature selection, and the results can be easily interpreted.
- Random Forest: Random forest is an ensemble learning technique that combines multiple decision trees to improve the classification performance. It is widely used in spam detection due to its high accuracy and robustness.
- Support Vector Machines: Support Vector Machines (SVMs) are a popular machine learning algorithm used for classification tasks. They work by finding the hyperplane that maximizes the margin between the different classes.
- Naïve Bayes: Naïve Bayes is a probabilistic algorithm widely used in text classification tasks, including spam detection. It works by calculating the probability of a message being spam given its features.
Conclusion
With the right tools and techniques, it is possible to build highly effective spam mail detection systems using machine learning. By leveraging the power of these techniques, we can help protect individuals and organizations from the growing threat of spam and other email-based attacks.
Frequently Asked Question(FAQs):
Q: Why is spam mail detection important?
Spam mail can contain malicious content, such as phishing scams or malware, which can harm individuals or organizations. By detecting and filtering out spam, we can reduce the risk of these attacks.
Q. What types of machine learning algorithms are used for spam mail detection?
Several machine learning algorithms can be used for spam mail detection, including decision trees, support vector machines, and neural networks.
Q. How can I evaluate the performance of my machine learning model for spam mail detection?
Performance metrics such as accuracy, precision, recall, and F1 score can be used to evaluate the performance of a machine learning model for spam mail detection. Cross-validation and confusion matrices can also be used for model evaluation.
Perfect eLearning is a tech-enabled education platform that provides IT courses with 100% Internship and Placement support. Perfect eLearning provides both Online classes and Offline classes only in Faridabad.
It provides a wide range of courses in areas such as Artificial Intelligence, Cloud Computing, Data Science, Digital Marketing, Full Stack Web Development, Block Chain, Data Analytics, and Mobile Application Development. Perfect eLearning, with its cutting-edge technology and expert instructors from Adobe, Microsoft, PWC, Google, Amazon, Flipkart, Nestle and Info edge is the perfect place to start your IT education.
Perfect eLearning provides the training and support you need to succeed in today's fast-paced and constantly evolving tech industry, whether you're just starting out or looking to expand your skill set.
There's something here for everyone. Perfect eLearning provides the best online courses as well as complete internship and placement assistance.
Keep Learning, Keep Growing.
If you are confused and need Guidance over choosing the right programming language or right career in the tech industry, you can schedule a free counselling session with Perfect eLearning experts.
In 2024, the number of global e-mail users is set to grow to 4.48 billion users, up from 3.8 billion in 2018. However, with the increase in email usage, there has also been a surge in the number of unwanted emails or spam mail. These unsolicited messages can be annoying, time-consuming, and pose a significant risk to cybersecurity. To tackle this issue, researchers have been developing techniques for spam mail detection, and one of the most promising approaches is using machine learning. Here we will discuss how machine learning techniques such as natural language processing, text classification, feature engineering, and different algorithms can help in spam mail detection using machine learning.
Several machine learning algorithms can be used for spam mail detection, including decision trees, support vector machines, and neural networks.